Text by: Dr Hatice Gunes, Leader of Affective Intelligence & Robotics Lab, Department of Computer Science and Technology, University of Cambridge
Recognition of expressions of emotions and affect from facial images is a well-studied research problem in the fields of affective computing and computer vision with a large number of datasets available containing facial images and corresponding expression labels [1]. Thanks to the unprecedented advances in machine learning field, many techniques for tackling this task now use deep learning approaches which require large datasets of facial images labelled with the expression or affect displayed. An important limitation of such a data-driven approach to affect recognition is being prone to biases in the datasets against certain demographic groups. The datasets that these algorithms are trained on do not necessarily contain an even distribution of subjects in terms of demographic attributes such as race, gender and age. Moreover, majority of the existing datasets that are made publicly available for research purposes do not contain information regarding these attributes, making it difficult to assess bias, let alone mitigate it. Machine learning models, unless explicitly modified, are severely impacted by such biases since they are given more opportunities (more training samples) for optimizing their objectives towards the majority group represented in the dataset. This leads to lower performances for the minority groups, i.e., subjects represented with less number of samples.
To address these issues, many solutions have been proposed in the machine learning community over the years, e.g. by addressing the problem at the data level with data generation or sampling approaches, at the feature level using adversarial learning or at the task level using multi-domain/task learning. Bias and mitigation strategies in facial analysis have attracted increasing attention both from the general public and the research communities. Many studies have investigated bias and mitigation strategies for face recognition, gender recognition, age estimation, kinship verification and face image quality estimation. However, studies specifically analysing, evaluating and mitigating race, gender and age biases in affect recognition have been scarce.
Therefore, in our recent research work [1] as part of the WorkingAge project, we undertook a systematic investigation of bias and fairness in facial expression. This is an important undertaking in the context of WorkingAge project because the average age of target user group is 45+, i.e. older than the average age of the datasets that are typically used for training predictive models for facial affect analysis. Therefore we need to understand whether and how the scarceness of expression data from older populations will impact our facia affect analysis module and the predictions it generates for the WAOW tool.
To this end, we considered three different approaches, namely a baseline deep network, an attribute-aware network and a representation-disentangling network under the two conditions of with and without data augmentation. Data augmentation refers to techniques used to increase the amount of data by adding slightly modified copies of already existing data or newly created synthetic data from existing data. In our attribute-aware solution, we provide a representation of the attributes as another input to the classification layer. This approach allows us to investigate how explicitly providing the attribute information can affect the expression recognition performance and whether it can mitigate bias. The main idea for the disentanglement approach is to make sure the learned representation does not contain any information about the sensitive attributes of race, gender and age.
With these three methods in place, we conducted experiments on RAF-DB [4] and CelebA [5] datasets that contain labels in terms of gender, age and/or race. To the best of our knowledge, ours is the first work (i) to perform an extensive analysis of bias and fairness for facial expression recognition, (ii) to use the sensitive attribute labels as input to the learning model to address bias, and (iii) to extend the disentanglement work of [3] to the area of facial expression recognition in order to learn fairer representations as a bias mitigation strategy.
The technical and experimental details of our study are provided in our research paper [1] and have been presented by Tia Xu at the of 16th European Conference on Computer Vision (ECCV 2020) Workshop on Fair Face Recognition and Analysis – http://chalearnlap.cvc.uab.es/workshop/37/description/.
In summary, what we found is that (i) augmenting the datasets improves the accuracy of the prediction model, but this alone is unable to mitigate the bias effect; (ii) both the attribute-aware and the disentangled approaches equipped with data augmentation perform better than the baseline approach in terms of accuracy and fairness; (iii) the disentangled approach is the best for mitigating demographic bias; and (iv) the bias mitigation strategies are more suitable in the existence of uneven attribute distribution or imbalanced number of subgroup data.
Below we provide a snapshot of Table 5 from our paper [1] to illustrate the challenging cases and qualitative results from the different models we have investigated.
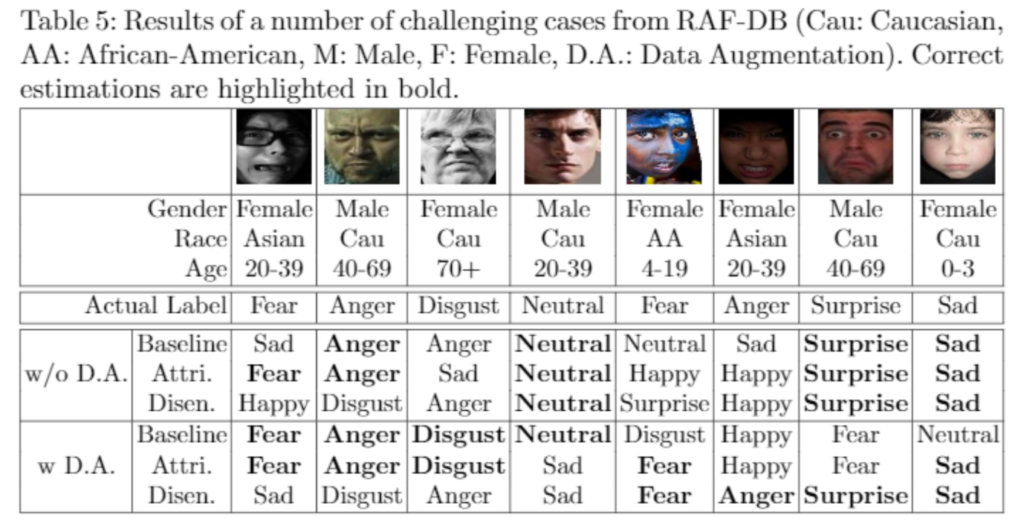
The most relevant finding for the WorkingAge project is that, for the age group of 40-65, data augmentation, attribute awareness and disentanglement help improve the accuracy of the machine learning models for facial expression recognition, and the disentangled approach provides fairer classification results. We will take these findings into account when finalising our facial affect analysis module to be integrated into the WAOW Tool.
References
[1] Sariyanidi, E., Gunes, H., Cavallaro, A.: Automatic analysis of facial affect:A survey of registration, representation, and recognition. IEEE Transactionson Pattern Analysis and Machine Intelligence37(6), 1113–1133 (June 2015).https://doi.org/10.1109/TPAMI.2014.2366127
[2] Xu, T., White, J., Kalkan, S. & Gunes, H. Investigating Bias and Fairness in Facial Expression Recognition, Proc. of 16th European Conference on Computer Vision (ECCV 2020) Workshop on Fair Face Recognition and Analysis, 2020. https://arxiv.org/abs/2007.10075
[3] Locatello, F., Abbati, G., Rainforth, T., Bauer, S., Scholkopf, B., Bachem, O.: Onthe fairness of disentangled representations. In: Advances in Neural InformationProcessing Systems. pp. 14611–14624 (2019)
[4] Li, S., Deng, W., Du, J.: Reliable crowdsourcing and deep locality-preserving learning for expression recognition in the wild. In: Proceedings of the IEEE conference on computer vision and pattern recognition. pp. 2852–2861 (2017)
[5] Liu, Z., Luo, P., Wang, X., Tang, X.: Deep learning face attributes in the wild. In: Proceedings of International Conference on Computer Vision (ICCV) (December2015)