Text by: Chaudhary Muhammad Aqdus Ilyas, University of Cambridge, UK
CARE 2020, an international Workshop on Pattern Recognition for positive teChnology And eldeRly wEllbeing (CARE 2020) was organized in conjunction with ICPR2020 – the 25th International Conference on Pattern Recognition, Milan, Italy, January 2021. The organizers were the principal investigators of the project “Stairway to elders: bridging space, time and emotions in their social environment for wellbeing“. The project aims to design methods for automatically evaluate the emotional wellbeing of elderly, involving innovative computational models and research techniques to measure the emotional state in an unconstrained, impartial and ecological way. The team contributes technical skills to achieve its main goal: designing and validating methods for continuous monitoring from multiple source of data of the emotional wellbeing and social interaction level of aging people, with strategic interventions from the environment to help them to get back both to positive emotions, in case of low-mood detection, and to a good level of social interaction [1].
Dr. Hatice Gunes and Dr. Andrea Gaggioli presented the keynote talks highlighting the importance of Artificial Emotional Intelligence for wellbeing and the role of Positive Technology in designing digital experience for positive change.
Dr. Hatice Gunes, a Reader ( Associate Professor ) and director of Affective Intelligence and Robotics (AFAR) at Cambridge University UK, presented on her groups’ work that aligns with the ethos of CARE 2020 conference, where she presented the various aspects of emotional intelligence that is the communication of non-verbal social signals, that are constantly emitted by senders and are also constantly interpreted by the receiving person or interface involving facial expressions, body language, gestures, and personal distance during social interactions, forming impressions and emotions. In short, the pipeline for creating systems with artificial emotional intelligence can be defined as expressions elicitation, attention regulation and drawing out a reaction from human users, the systems themselves then expressing emotions and social signals that can be identified by humans, and finally systems automatically detecting emotions and social signals of humans. Such systems can be considered as having Artificial Emotional Intelligence (AEI).
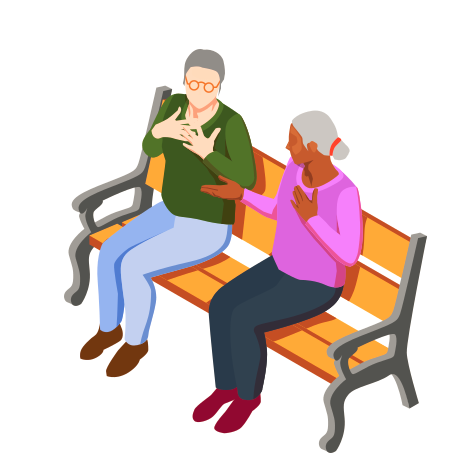
Emotional wellbeing together with health, is a key factor for aging well. Social interaction is a key aspect influencing the wellbeing of elderly’s life. Its lack may produce cognitive deconstruction, which includes emotion suppression, altered sense of time, poor planned actions and mobility, decline of meaningful thought [1].
Dr. Hatice Gunes presented the number of applications of AEI, for instance, creating a Sensitive Artificial Listeners (SAL), an European Union (EU) project, that uses Communication-driven Model. SAL is the first fully automatic system that engages humans in the sustained conversational interaction based on non-verbal behavior interpretation [2].
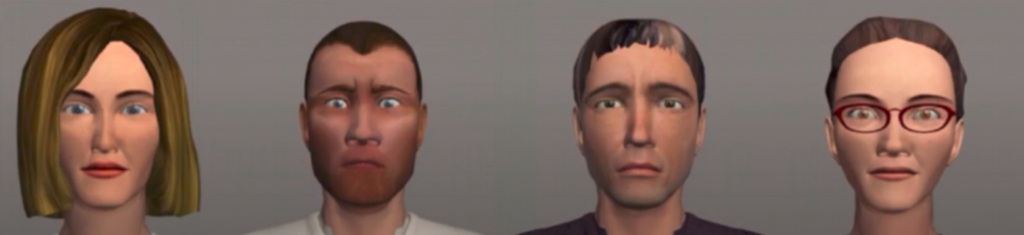
The four SAL agents (from left to right): Poppy, Spike, Obadiah and Prudence [2]
This project focused on the two modalities of vision and audio where the user is sensed through camera and microphone, followed by the feature extraction and feature fusion. Interpreter receives the consolidated analyses to understand the user state, agent state and dialogue state and generate the appropriate action of the virtual agent based on user expressions. These virtual agents equipped with AEI can be used for healthcare support in the clinical setting such as SimSensei [3].
Dr. Hatice Gunes demonstrated another related work regarding development of AEI systems based on data driven models for recognition and prediction of expressions and performance evaluation by context sensitive interpretation of emotional cues. This study involves 30 participants who played a custom video game Memory Break in two environmental settings; Desktop and Virtual Reality (VR). The Working Memory (WM) capacity baseline of participants were measured using the Automated Operation Span Task [5] along with self-reported affective states and filled the In-Game Experience Questionnaire [6]. The participants were divided into two groups based on their WM capacity baseline scores; high-WM and low-WM. Performance is evaluated using VR and Desktop settings and it is observed that participants with low-WM had a significantly better WM performance in VR compared to Desktop settings. It is also observed that Valence is also significantly correlated with WM performance [7]. It is observed that participants achieve their best WM performance when both valence and arousal are high, that is when participants are in flow state (state of enjoyment) as we have mentioned in our previous blog.
Dr. Hatice Gunes concluded the talk by presenting the WorkingAge project and the aspects in the project that relate to AEI.
In conclusion, Dr. Hatice Gunes highlighted the importance of artificial emotional intelligence when creating assistive technology for wellbeing and discussed the challenges related to developing emotional intelligent systems in broader contexts.
References:
- “Stairway to elders”, Phuselab.di.unimi.it, 2021. [Online]. Available: http://phuselab.di.unimi.it/S2E/index.html. [Accessed: 10- Mar- 2021].
- M. Schröder et al., “Building autonomous sensitive artificial listeners (Extended abstract),” 2015 International Conference on Affective Computing and Intelligent Interaction (ACII), Xi’an, China, 2015, pp. 456-462, doi: 10.1109/ACII.2015.7344610.
- DeVault, D., Artstein, R., Benn, G., Dey, T., Fast, E., Gainer, A., … & Morency, L. P. (2014, May). SimSensei Kiosk: A virtual human interviewer for healthcare decision support. In Proceedings of the 2014 international conference on Autonomous agents and multi-agent systems (pp. 1061-1068).
- Reidy, L., Chan, D., Nduka, C., & Gunes, H. (2020, October). Facial Electromyography-based Adaptive Virtual Reality Gaming for Cognitive Training. In Proceedings of the 2020 International Conference on Multimodal Interaction (pp. 174-183).
- Foster, J. L., Shipstead, Z., Harrison, T. L., Hicks, K. L., Redick, T. S., & Engle, R. W. (2015). Shortened complex span tasks can reliably measure working memory capacity. Memory & cognition, 43(2), 226-236.
- IJsselsteijn, W. A., de Kort, Y. A., & Poels, K. (2013). The game experience questionnaire. Eindhoven: Technische Universiteit Eindhoven, 46(1).
- Gabana, D., Tokarchuk, L., Hannon, E., & Gunes, H. (2017, October). Effects of valence and arousal on working memory performance in virtual reality gaming. In 2017 Seventh International Conference on Affective Computing and Intelligent Interaction (ACII) (pp. 36-41).